Explanatory Spatial Analysis of Urban Traffic Crashes by Developing Semi-Parametric Geographically Weighted Poisson Regression

Abstract
The increasing road construction along with the increase in the number of vehicles in cities has enormously increased the number and severity of urban crashes. Therefore, developing innovative, robust models for the aim of crash prediction has been the concern of transportation specialists for so long. Global regression models with assuming Poisson or Negative Binomial distribution for errors describe the relationships between crashes and independent variables through estimating fixed coefficients which do not vary over the study area. Considering the spatial nature of crash data as well as its relating factors, actual spatial patterns might vary with local site conditions, which is also recognized as spatial non-stationarity. Accordingly, Semi parametric geographically weighted Poisson regression (S-GWPR) is employed for crash count data aggregated on traffic analysis zones (TAZs) in Mashhad, Iran, to capture the spatial heterogeneity that exists in the relationship between crash counts and explanatory variables over the study area. Then the global Poisson model and S-GWPR are compared by the measures of goodness of fit such as the correlation coefficient, percent deviance explained (PDE), AICc, and MSE to identify whether the new model indicates any improvement over the global one. Moran’s I indicator has been carried out on residuals of the two models to compare the ability of each model in addressing spatial autocorrelation. The results revealed that S-GWPR not only represents a significant improvement of model performance over the global model indicated, but also has successfully addressed the spatial autocorrelation of residuals. Furthermore, according to the results of local coefficients estimated for different network variables used in the model, regions with higher priority of improvement measures for different road types can be identified.
Results
As can be seen in the below tables, the proposed S-GWPR model outperformed the traditional Poisson model in terms of AICc and BIC. Also, the local model has been successful in capturing 75% of deviance in data, while the global model has explained only 51% of the deviance.
Also, The estimated coefficients resulting from S-GWPR are examined to investigate for the spatial non-stationary state. The results are summarized in Table 3. Accordingly, it can be infered that the process modeled is spatially non-stationary for all variables.
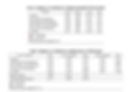
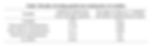
Although the estimated coefficients of "total freeway road kilometers" are significant for both global and mixed models as indicated in Table 1 and Table 2, the magnitude of coefficients are not the same. Another interesting point is that the estimated coefficients for the rest of variables in global model and the corresponding mean values resulting from S-GWPR are almost similar in terms of magnitude and sign.
Based on the figure below, it can be inferred that local estimated coefficients for "total arterial road kilometers" are positive in most of TAZs (areas indicated by red, orange, yellow and light green colors). However, several areas with negative coefficients can be found in northeast parts of the study area (areas indicated by darker green color).
​
It is worth to note that the intensity of the relationships between crash counts and “total arterial road kilometers” is not constant across the study area. It is in contrast to the results of global Poisson model which assume a constant parameter for the variables. The direct and stronger relationships are found in west, southeast and several regions in north part of study area. In such areas, the effect of "total arterial road kilometers" upon crash occurrences is relatively higher than other TAZs. The varying influence of "total arterial road kilometers" upon crash occurrence in Mashhad can be explained through the two main city entrance and exit gates locating in southeast and northwest of Mashhad. The greater effect of “total arterial road kilometers” on crash occurrences in these areas could be related to the sudden changes in the environment and the speed that the drivers experience when entering the city.
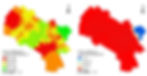
Local Percent Deviance Explained
Percent deviance explained (PDE) measures the proportion of deviance in the observed data explained by the model. Local variations of (PDE) statistic can be computed to give a sense for how well a local model can replicate the data recorded in the vicinity of the regression point. In addition to local coefficients, (PDE) provides an appropriate way to explore the spatial heterogeneity and varying relationships as well.
​
Unlike the global model, the spatial patterns of local (PDE) in S-GWPR represent a marked regional differentiation. Local (PDE) is characterized by higher values (0.74-0.83) in the southeast and some central parts of the study area (the following figure). Accordingly, it can be seen that the model did not fit very well to data for TAZs located in eastern part of Mashhad. This figure helps to realize additional explanatory factors were required and where could those factors be applied.
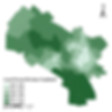